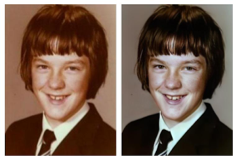
Bringing Old Photos Back to Life
Recreating results of the paper "Bringing Old Photos Back to Life" by implementing VAE, Pix2pix and CycleGAN.
Abstract: Photos are taken to freeze the happy moments that otherwise are gone. Old photo prints deteriorate when kept in poor environmental condition, which causes the valuable photo content to be permanently damaged. The paper, “Bringing old photos back to life”, proposes to restore old photos that suffer from severe degradation through a deep learning approach. The domain gap between synthetic images and real old photos makes the network fail to generalize. This paper proposes a novel triplet domain translation network by leveraging real photos along with massive synthetic image pairs. It trains two variational autoencoders (VAEs) to respectively transform old photos and clean photos into two latent spaces. This translation generalizes well to real photos because the domain gap is closed in the compact latent space. In our project, we make an attempt to implement this paper in order to restore the old images. In addition to this, implementation of other deep learning architectures such as Pix2Pix and CycleGAN is done for further analysis.